In these scenarios, a deep-learning machine is given the rules of the game and then plays against itself. Crucially, it is rewarded at each step according to how it performs. This reward process is hugely important because it helps the machine to distinguish good play from bad play. In other words, it helps the machine learn.
But this doesn’t work in many real-world situations, because rewards are often rare or hard to determine.
Recommended for You
For example, random turns of a Rubik’s Cube cannot easily be rewarded, since it is hard to judge whether the new configuration is any closer to a solution. And a sequence of random turns can go on for a long time without reaching a solution, so the end-state reward can only be offered rarely.
In chess, by contrast, there is a relatively large search space but each move can be evaluated and rewarded accordingly. That just isn’t the case for the Rubik’s Cube.
Enter Stephen McAleer and colleagues from the University of California, Irvine. These guys have pioneered a new kind of deep-learning technique, called “autodidactic iteration,” that can teach itself to solve a Rubik’s Cube with no human assistance. The trick that McAleer and co have mastered is to find a way for the machine to create its own system of rewards.
Here’s how it works. Given an unsolved cube, the machine must decide whether a specific move is an improvement on the existing configuration. To do this, it must be able to evaluate the move.
Autodidactic iteration does this by starting with the finished cube and working backwards to find a configuration that is similar to the proposed move. This process is not perfect, but deep learning helps the system figure out which moves are generally better than others.
Having been trained, the network then uses a standard search tree to hunt for suggested moves for each configuration.
The result is an algorithm that performs remarkably well. “Our algorithm is able to solve 100% of randomly scrambled cubes while achieving a median solve length of 30 moves—less than or equal to solvers that employ human domain knowledge,” say McAleer and co.
That’s interesting because it has implications for a variety of other tasks that deep learning has struggled with, including puzzles like Sokoban, games like Montezuma’s Revenge, and problems like prime number factorization.
Indeed, McAleer and co have other goals in their sights: “We are working on extending this method to find approximate solutions to other combinatorial optimization problems such as prediction of protein tertiary structure.”
Source: A machine has figured out Rubik’s Cube all by itself – MIT Technology Review
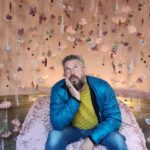
Robin Edgar
Organisational Structures | Technology and Science | Military, IT and Lifestyle consultancy | Social, Broadcast & Cross Media | Flying aircraft