Human-generated knowledge bases like Wikipedia have a recall problem. First, there are the articles that should be there but are entirely missing. The unknown unknowns.
Consider Joelle Pineau, the Canadian roboticist bringing scientific rigor to artificial intelligence and who directs Facebook’s new AI Research lab in Montreal. Or Miriam Adelson, an actively publishing addiction treatment researcher who happens to be a billionaire by marriage and a major funder of her own field. Or Evelyn Wang, the new head of MIT’s revered MechE department whose accomplishments include a device that generates drinkable water from sunlight and desert air. When I wrote this a few days ago, none of them had articles on English Wikipedia, though they should by any measure of notability.
(Pineau is up now thanks to my friend and fellow science crusader Jess Wade who created an article just hours after I told her about Pineau’s absence. And if the internet is in a good mood, someone will create articles for the other two soon after this post goes live.)
But I didn’t discover those people on my own. I used a machine learning system we’re building at Primer. It discovered and described them for me. It does this much as a human would, if a human could read 500 million news articles, 39 million scientific papers, all of Wikipedia, and then write 70,000 biographical summaries of scientists.
[…]
We are publicly releasing free-licensed data about scientists that we’ve been generating along the way, starting with 30,000 computer scientists. Only 15% of them are known to Wikipedia. The data set includes 1 million news sentences that quote or describe the scientists, metadata for the source articles, a mapping to their published work in the Semantic Scholar Open Research Corpus, and mappings to their Wikipedia and Wikidata entries. We will revise and add to that data as we go. (Many thanks to Oren Etzioni and AI2 for data and feedback.) Our aim is to help the open data research community build better tools for maintaining Wikipedia and Wikidata, starting with scientific content.
Fluid Knowledge
We trained Quicksilver’s models on 30,000 English Wikipedia articles about scientists, their Wikidata entries, and over 3 million sentences from news documents describing them and their work. Then we fed in the names and affiliations of 200,000 authors of scientific papers.
In the morning we found 40,000 people missing from Wikipedia who have a similar distribution of news coverage as those who do have articles. Quicksilver doubled the number of scientists potentially eligible for a Wikipedia article overnight.
It also revealed the second flavor of the recall problem that plagues human-generated knowledge bases: information decay. For most of those 30,000 scientists who are on English Wikipedia, Quicksilver identified relevant information that was missing from their articles.
Source: Primer | Machine-Generated Knowledge Bases
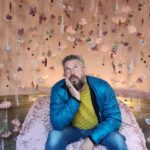
Robin Edgar
Organisational Structures | Technology and Science | Military, IT and Lifestyle consultancy | Social, Broadcast & Cross Media | Flying aircraft