Now that DeepMind has solved Go, the company is applying DeepMind to navigation. Navigation relies on knowing where you are in space relative to your surroundings and continually updating that knowledge as you move. DeepMind scientists trained neural networks to navigate like this in a square arena, mimicking the paths that foraging rats took as they explored the space. The networks got information about the rat’s speed, head direction, distance from the walls, and other details. To researchers’ surprise, the networks that learned to successfully navigate this space had developed a layer akin to grid cells. This was surprising because it is the exact same system that mammalian brains use to navigate.
A few different cell populations in our brains help us make our way through space. Place cells are so named because they fire when we pass through a particular place in our environment relative to familiar external objects. They are located in the hippocampus—a brain region responsible for memory formation and storage—and are thus thought to provide a cellular place for our memories. Grid cells got their name because they superimpose a hypothetical hexagonal grid upon our surroundings, as if the whole world were overlaid with vintage tiles from the floor of a New York City bathroom. They fire whenever we pass through a node on that grid.
More DeepMind experiments showed that only the neural networks that developed layers that “resembled grid cells, exhibiting significant hexagonal periodicity (gridness),” could navigate more complicated environments than the initial square arena, like setups with multiple rooms. And only these networks could adjust their routes based on changes in the environment, recognizing and using shortcuts to get to preassigned goals after previously closed doors were opened to them.
Implications
These results have a couple of interesting ramifications. One is the suggestion that grid cells are the optimal way to navigate. They didn’t have to emerge here—there was nothing dictating their formation—yet this computer system hit upon them as the best solution, just like our biological system did. Since the evolution of any system, cell type, or protein can proceed along multiple parallel paths, it is very much not a given that the system we end up with is in any way inevitable or optimized. This report seems to imply that, with grid cells, that might actually be the case.
Another implication is the support for the idea that grid cells function to impose a Euclidian framework upon our surroundings, allowing us to find and follow the most direct route to a (remembered) destination. This function had been posited since the discovery of grid cells in 2005, but it had not yet been proven empirically. DeepMind’s findings provide a biological bolster for the idea floated by Kant in the 18th century that our perception of place is an innate ability, independent of experience.
Source: AI trained to navigate develops brain-like location tracking | Ars Technica
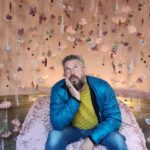
Robin Edgar
Organisational Structures | Technology and Science | Military, IT and Lifestyle consultancy | Social, Broadcast & Cross Media | Flying aircraft