In March, Yoshua Bengio received a share of the Turing Award, the highest accolade in computer science, for contributions to the development of deep learning—the technique that triggered a renaissance in artificial intelligence, leading to advances in self-driving cars, real-time speech translation, and facial recognition.
Now, Bengio says deep learning needs to be fixed. He believes it won’t realize its full potential, and won’t deliver a true AI revolution, until it can go beyond pattern recognition and learn more about cause and effect. In other words, he says, deep learning needs to start asking why things happen.
[…]
Machine learning systems including deep learning are highly specific, trained for a particular task, like recognizing cats in images, or spoken commands in audio. Since bursting onto the scene around 2012, deep learning has demonstrated a particularly impressive ability to recognize patterns in data; it’s been put to many practical uses, from spotting signs of cancer in medical scans to uncovering fraud in financial data.
But deep learning is fundamentally blind to cause and effect. Unlike a real doctor, a deep learning algorithm cannot explain why a particular image may suggest disease. This means deep learning must be used cautiously in critical situations.
[…]
At his research lab, Bengio is working on a version of deep learning capable of recognizing simple cause-and-effect relationships. He and colleagues recently posted a research paper outlining the approach. They used a dataset that maps causal relationships between real-world phenomena, such as smoking and lung cancer, in terms of probabilities. They also generated synthetic datasets of causal relationships.
[…]
Others believe the focus on deep learning may be part of the problem. Gary Marcus, a professor emeritus at NYU and the author of a recent book that highlights the limits of deep learning, Rebooting AI: Building Artificial Intelligence We Can Trust, says Bengio’s interest in causal reasoning signals a welcome shift in thinking.
“Too much of deep learning has focused on correlation without causation, and that often leaves deep learning systems at a loss when they are tested on conditions that aren’t quite the same as the ones they were trained on,” he says.
Marcus adds that the lesson from human experience is obvious. “When children ask ‘why?’ they are asking about causality,” he says. “When machines start asking why, they will be a lot smarter.”
Source: An AI Pioneer Wants His Algorithms to Understand the ‘Why’ | WIRED
This is a hugely important – and old – question in this field. Without the ‘why’, humans must ‘just trust’ answers given by AI that seem intuitively strange. When you’re talking about health care or human related activities such as liability ‘just accept what I’m telling you’ isn’t good enough.
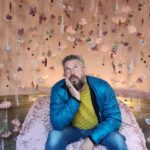
Robin Edgar
Organisational Structures | Technology and Science | Military, IT and Lifestyle consultancy | Social, Broadcast & Cross Media | Flying aircraft