We’re delighted to announce you can now take advantage of graph-native machine learning (ML) inside of Neo4j! We’ve just released a preview of Neo4j’s Graph Data Science™ Library version 1.4, which includes graph embeddings and an ML model catalog.
Together, these enable you to create representations of your graph and make graph predictions – all within Neo4j.
[…]
Graph Embeddings
The graph embedding algorithms are the star of the show in this release.
These algorithms are used to transform the topology and features of your graph into fixed-length vectors (or embeddings) that uniquely represent each node.
Graph embeddings are powerful, because they preserve the key features of the graph while reducing dimensionality in a way that can be decoded. This means you can capture the complexity and structure of your graph and transform it for use in various ML predictions.
Graph embeddings capture the nuances of graphs in a way that can be used to make predictions or lower dimensional visualizations.
In this release, we are offering three embedding options that learn the graph topology and, in some cases, node properties to calculate more accurate representations:
- This is probably the most well-known graph embedding algorithm. It uses random walks to sample a graph, and a neural network to learn the best representation of each node.
- A more recent graph embedding algorithm that uses linear algebra to project a graph into lower dimensional space. In GDS 1.4, we’ve extended the original implementation to support node features and directionality as well.
- FastRP is up to 75,000 times faster than Node2Vec, while providing equivalent accuracy!
- This is an embedding technique using inductive representation learning on graphs, via graph convolutional neural networks, where the graph is sampled to learn a function that can predict embeddings (rather than learning embeddings directly). This means you can learn on a subset of your graph and use that representative function for new data and make continuous predictions as your graph updates. (Wow!)
- If you’d like a deeper dive into how it works, check out the GraphSAGE session from the NODES event.
Graph embeddings available in the Neo4j Graph Data Science Library v1.4 . The caution marks indicate that, while directions are supported, our internal benchmarks don’t show performance improvements.
Graph ML Model Catalog
GraphSAGE trains a model to predict node embeddings for unseen parts of the graph, or new data as mentioned above.
To really capitalize on what GraphSAGE can do, we needed to add a catalog to be able to store and reference these predictive models. This model catalog lives in the Neo4j analytics workspace and contains versioning information (what data was this trained on?), time stamps and, of course, the model names.
When you want to use a model, you can provide the name of the model to GraphSAGE, along with the named graph you want to apply it to.
GraphSAGE ML Models are stored in the Neo4j analytics workspace.
[…]
Source: Announcing: Graph-Native Machine Learning in Neo4j!
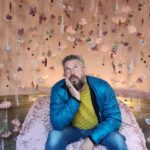
Robin Edgar
Organisational Structures | Technology and Science | Military, IT and Lifestyle consultancy | Social, Broadcast & Cross Media | Flying aircraft