Known as SEER, short for SElf-supERvised, this massive convolutional neural network contains over a billion parameters. If you show it images of things, it will describe in words what it recognizes: a bicycle, a banana, a red-and-blue striped golfing umbrella, and so on. While its capabilities aren’t all that novel, the way it was trained differs from the techniques used to teach other types of computer vision models. Essentially, SEER partly taught itself using an approach called self-supervision.
First, it learned how to group the Instagram pictures by their similarity without any supervision, using an algorithm nicknamed SwAV. The team then fine-tuned the model by teaching it to associate a million photos taken from the ImageNet dataset with their corresponding human-written labels. This stage was a traditional supervised method: humans curated the photos and labels, and this is passed on to the neural network that was pretrained by itself.
[…]
“SwAV uses online clustering to rapidly group images with similar visual concepts and leverage their similarities. With SwAV, we were able to improve over the previous state of the art in self-supervised learning — and did so with 6x less training time.”
SEER thus learned to associate an image of, say, a red apple with the description “red apple.” Once trained, the model’s object-recognition skills were tested using 50,000 pictures from ImageNet it had not seen before: in each test it had to produce a set of predictions of what was pictured, ranked in confidence from high to low. Its top prediction in each test was accurate 84.2 per cent of time, we’re told.
The model doesn’t score as highly as its peers in ImageNet benchmarking. The downside of models like SEER is that they’re less accurate than their supervised cousins. Yet there are advantages to training in a semi-supervised way, Goyal, first author of the project’s paper on SEER, told The Register.
“Using self-supervision pretraining, we can learn on a more diverse set of images as we don’t require labels, data curation or any other metadata,” she said. “This means that the model can learn about more visual concepts in the world in contrast to the supervised training where we can only train on limited or small datasets that are highly curated and don’t allow us to capture visual diversity of the world.”
[…]
SEER was trained over eight days using 512 GPUs. The code for the model isn’t publicly available, although VISSL, the PyTorch library that was used to build SEER, is now up on GitHub.
[…]
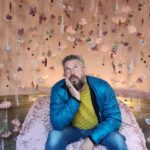
Robin Edgar
Organisational Structures | Technology and Science | Military, IT and Lifestyle consultancy | Social, Broadcast & Cross Media | Flying aircraft