Google has announced a new Explainable AI feature for its cloud platform, which provides more information about the features that cause an AI prediction to come up with its results.
Artificial neural networks, which are used by many of today’s machine learning and AI systems, are modelled to some extent on biological brains. One of the challenges with these systems is that as they have become larger and more complex, it has also become harder to see the exact reasons for specific predictions. Google’s white paper on the subject refers to “loss of debuggability and transparency”.
The uncertainty this introduces has serious consequences. It can disguise spurious correlations, where the system picks on an irrelevant or unintended feature in the training data. It also makes it hard to fix AI bias, where predictions are made based on features that are ethically unacceptable.
AI Explainability has not been invented by Google but is widely researched. The challenge is how to present the workings of an AI system in a form which is easily intelligible.
Google has come up with a set of three tools under this heading of “AI Explainability” that may help. The first and perhaps most important is AI Explanations, which lists features detected by the AI along with an attribution score showing how much each feature affected the prediction. In an example from the docs, a neural network predicts the duration of a bike ride based on weather data and previous ride information. The tool shows factors like temperature, day of week and start time, scored to show their influence on the prediction.
In the case of images, an overlay shows which parts of the picture were the main factors in the classification of the image content.
There is also a What-If tool that lets you test model performance if you manipulate individual attributes, and a continuous evaluation tool that feeds sample results to human reviewers on a schedule to assist monitoring of results.
AI Explainability is useful for evaluating almost any model and near-essential for detecting bias, which Google considers part of its approach to responsible AI.
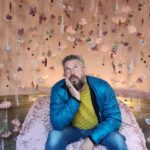
Robin Edgar
Organisational Structures | Technology and Science | Military, IT and Lifestyle consultancy | Social, Broadcast & Cross Media | Flying aircraft