Jiaming Luo and Regina Barzilay from MIT and Yuan Cao from Google’s AI lab in Mountain View, California. This team has developed a machine-learning system capable of deciphering lost languages, and they’ve demonstrated it by having it decipher Linear B—the first time this has been done automatically. The approach they used was very different from the standard machine translation techniques.
First some background. The big idea behind machine translation is the understanding that words are related to each other in similar ways, regardless of the language involved.
So the process begins by mapping out these relations for a specific language. This requires huge databases of text. A machine then searches this text to see how often each word appears next to every other word. This pattern of appearances is a unique signature that defines the word in a multidimensional parameter space. Indeed, the word can be thought of as a vector within this space. And this vector acts as a powerful constraint on how the word can appear in any translation the machine comes up with.
These vectors obey some simple mathematical rules. For example: king – man + woman = queen. And a sentence can be thought of as a set of vectors that follow one after the other to form a kind of trajectory through this space.
The key insight enabling machine translation is that words in different languages occupy the same points in their respective parameter spaces. That makes it possible to map an entire language onto another language with a one-to-one correspondence.
In this way, the process of translating sentences becomes the process of finding similar trajectories through these spaces. The machine never even needs to “know” what the sentences mean.
This process relies crucially on the large data sets. But a couple of years ago, a German team of researchers showed how a similar approach with much smaller databases could help translate much rarer languages that lack the big databases of text. The trick is to find a different way to constrain the machine approach that doesn’t rely on the database.
Now Luo and co have gone further to show how machine translation can decipher languages that have been lost entirely. The constraint they use has to do with the way languages are known to evolve over time.
The idea is that any language can change in only certain ways—for example, the symbols in related languages appear with similar distributions, related words have the same order of characters, and so on. With these rules constraining the machine, it becomes much easier to decipher a language, provided the progenitor language is known.
Luo and co put the technique to the test with two lost languages, Linear B and Ugaritic. Linguists know that Linear B encodes an early version of ancient Greek and that Ugaritic, which was discovered in 1929, is an early form of Hebrew.
Given that information and the constraints imposed by linguistic evolution, Luo and co’s machine is able to translate both languages with remarkable accuracy. “We were able to correctly translate 67.3% of Linear B cognates into their Greek equivalents in the decipherment scenario,” they say. “To the best of our knowledge, our experiment is the first attempt of deciphering Linear B automatically.”
That’s impressive work that takes machine translation to a new level. But it also raises the interesting question of other lost languages—particularly those that have never been deciphered, such as Linear A.
In this paper, Linear A is conspicuous by its absence. Luo and co do not even mention it, but it must loom large in their thinking, as it does for all linguists. Yet significant breakthroughs are still needed before this script becomes amenable to machine translation.
For example, nobody knows what language Linear A encodes. Attempts to decipher it into ancient Greek have all failed. And without the progenitor language, the new technique does not work.
But the big advantage of machine-based approaches is that they can test one language after another quickly without becoming fatigued. So it’s quite possible that Luo and co might tackle Linear A with a brute-force approach—simply attempt to decipher it into every language for which machine translation already operates.
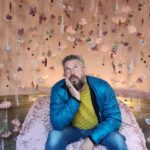
Robin Edgar
Organisational Structures | Technology and Science | Military, IT and Lifestyle consultancy | Social, Broadcast & Cross Media | Flying aircraft