Why did the frog cross the road? Well, a new artificial intelligent (AI) agent that can play the classic arcade game Frogger not only can tell you why it crossed the road, but it can justify its every move in everyday language.
Developed by Georgia Tech, in collaboration with Cornell and the University of Kentucky, the work enables an AI agent to provide a rationale for a mistake or errant behavior, and to explain it in a way that is easy for non-experts to understand.
This, the researchers say, may help robots and other types of AI agents seem more relatable and trustworthy to humans. They also say their findings are an important step toward a more transparent, human-centered AI design that understands people’s preferences and prioritizes people’s needs.
“If the power of AI is to be democratized, it needs to be accessible to anyone regardless of their technical abilities,” said Upol Ehsan, Ph.D. student in the School of Interactive Computing at Georgia Tech and lead researcher.
“As AI pervades all aspects of our lives, there is a distinct need for human-centered AI design that makes black-boxed AI systems explainable to everyday users. Our work takes a formative step toward understanding the role of language-based explanations and how humans perceive them.”
The study was supported by the Office of Naval Research (ONR).
Researchers developed a participant study to determine if their AI agent could offer rationales that mimicked human responses. Spectators watched the AI agent play the videogame Frogger and then ranked three on-screen rationales in order of how well each described the AI’s game move.
Of the three anonymized justifications for each move – a human-generated response, the AI-agent response, and a randomly generated response – the participants preferred the human-generated rationales first, but the AI-generated responses were a close second.
Frogger offered the researchers the chance to train an AI in a “sequential decision-making environment,” which is a significant research challenge because decisions that the agent has already made influence future decisions. Therefore, explaining the chain of reasoning to experts is difficult, and even more so when communicating with non-experts, according to researchers.
[…]
By a 3-to-1 margin, participants favored answers that were classified in the “complete picture” category. Responses showed that people appreciated the AI thinking about future steps rather than just what was in the moment, which might make them more prone to making another mistake. People also wanted to know more so that they might directly help the AI fix the errant behavior.
[…]
The research was presented in March at the Association for Computing Machinery’s Intelligent User Interfaces 2019 Conference. The paper is titled Automated Rationale Generation: A Technique for Explainable AI and its Effects on Human Perceptions. Ehsan will present a position paper highlighting the design and evaluation challenges of human-centered Explainable AI systems at the upcoming Emerging Perspectives in Human-Centered Machine Learning workshop at the ACM CHI 2019 conference, May 4-9, in Glasgow, Scotland.
Source: Research Findings May Lead to More Explainable AI | College of Computing
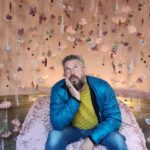
Robin Edgar
Organisational Structures | Technology and Science | Military, IT and Lifestyle consultancy | Social, Broadcast & Cross Media | Flying aircraft