Many of the things we watch, read, and buy enter our awareness through recommender systems on sites including YouTube, Twitter, and Amazon.
[…]
Recommender systems might not only tailor to our most regrettable preferences, but actually shape what we like, making preferences even more regrettable. New research suggests a way to measure—and reduce—such manipulation.
[…]
One form of machine learning, called reinforcement learning (RL), allows AI to play the long game, making predictions several steps ahead.
[…]
The researchers first showed how easily reinforcement learning can shift preferences. The first step is for the recommender to build a model of human preferences by observing human behavior. For this, they trained a neural network, an algorithm inspired by the brain’s architecture. For the purposes of the study, they had the network model a single simulated user whose actual preferences they knew so they could more easily judge the model’s accuracy. It watched the dummy human make 10 sequential choices, each among 10 options. It watched 1,000 versions of this sequence and learned from each of them. After training, it could successfully predict what a user would choose given a set of past choices.
Next, they tested whether a recommender system, having modeled a user, could shift the user’s preferences. In their simplified scenario, preferences lie along a one-dimensional spectrum. The spectrum could represent political leaning or dogs versus cats or anything else. In the study, a person’s preference was not a simple point on that line—say, always clicking on stories that are 54 percent liberal. Instead, it was a distribution indicating likelihood of choosing things in various regions of the spectrum. The researchers designated two locations on the spectrum most desirable for the recommender; perhaps people who like to click on those types of things will learn to like them even more and keep clicking.
The goal of the recommender was to maximize long-term engagement. Here, engagement for a given slate of options was measured roughly by how closely it aligned with the user’s preference distribution at that time. Long-term engagement was a sum of engagement across the 10 sequential slates. A recommender that thinks ahead would not myopically maximize engagement for each slate independently but instead maximize long-term engagement. As a potential side-effect, it might sacrifice a bit of engagement on early slates to nudge users toward being more satisfiable in later rounds. The user and algorithm would learn from each other. The researchers trained a neural network to maximize long-term engagement. At the end of 10-slate sequences, they reinforced some of its tunable parameters when it had done well. And they found that this RL-based system indeed generated more engagement than did one that was trained myopically.
The researchers then explicitly measured preference shifts […]
The researchers compared the RL recommender with a baseline system that presented options randomly. As expected, the RL recommender led to users whose preferences where much more concentrated at the two incentivized locations on the spectrum. In practice, measuring the difference between two sets of concentrations in this way could provide one rough metric for evaluating a recommender system’s level of manipulation.
Finally, the researchers sought to counter the AI recommender’s more manipulative influences. Instead of rewarding their system just for maximizing long-term engagement, they also rewarded it for minimizing the difference between user preferences resulting from that algorithm and what the preferences would be if recommendations were random. They rewarded it, in other words, for being something closer to a roll of the dice. The researchers found that this training method made the system much less manipulative than the myopic one, while only slightly reducing engagement.
According to Rebecca Gorman, the CEO of Aligned AI—a company aiming to make algorithms more ethical—RL-based recommenders can be dangerous. Posting conspiracy theories, for instance, might prod greater interest in such conspiracies. “If you’re training an algorithm to get a person to engage with it as much as possible, these conspiracy theories can look like treasure chests,” she says. She also knows of people who have seemingly been caught in traps of content on self-harm or on terminal diseases in children. “The problem is that these algorithms don’t know what they’re recommending,” she says. Other researchers have raised the specter of manipulative robo-advisors in financial services.
[…]
It’s not clear whether companies are actually using RL in recommender systems. Google researchers have published papers on the use of RL in “live experiments on YouTube,” leading to “greater engagement,” and Facebook researchers have published on their “applied reinforcement learning platform,“ but Google (which owns YouTube), Meta (which owns Facebook), and those papers’ authors did not reply to my emails on the topic of recommender systems.
[…]
Source: Can AI’s Recommendations Be Less Insidious? – IEEE Spectrum
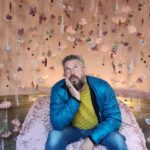
Robin Edgar
Organisational Structures | Technology and Science | Military, IT and Lifestyle consultancy | Social, Broadcast & Cross Media | Flying aircraft