Debates around the effectiveness of high-profile Twitter account suspensions and similar bans on abusive users across social media platforms abound. Yet we know little about the effectiveness of warning a user about the possibility of suspending their account as opposed to outright suspensions in reducing hate speech. With a pre-registered experiment, we provide causal evidence that a warning message can reduce the use of hateful language on Twitter, at least in the short term. We design our messages based on the literature on deterrence, and test versions that emphasize the legitimacy of the sender, the credibility of the message, and the costliness of being suspended. We find that the act of warning a user of the potential consequences of their behavior can significantly reduce their hateful language for one week. We also find that warning messages that aim to appear legitimate in the eyes of the target user seem to be the most effective. In light of these findings, we consider the policy implications of platforms adopting a more aggressive approach to warning users that their accounts may be suspended as a tool for reducing hateful speech online.
[…]
we test whether warning users of their potential suspension if they continue using hateful language might be able to reduce online hate speech. To do so, we implemented a pre-registered experiment on Twitter in order to test the ability of “warning messages” about the possibility of future suspensions to reduce hateful language online. More specifically, we identify users who are candidates for suspension in the future based on their prior tweets and download their follower lists before the suspension takes place. After a user gets suspended, we randomly assign some of their followers who have also used hateful language to receive a warning that they, too, may be suspended for the same reason.
Since our tweets aim to deter users from using hateful language, we design them relying on the three mechanisms that the literature on deterrence deems as most effective in reducing deviation behavior: costliness, legitimacy, and credibility. In other words, our experiment allows us to manipulate the degree to which users perceive their suspension as costly, legitimate, and credible.
[…]
Our study provides causal evidence that the act of sending a warning message to a user can significantly decrease their use of hateful language as measured by their ratio of hateful tweets over their total number of tweets. Although we do not find strong evidence that distinguishes between warnings that are high versus low in legitimacy, credibility, or costliness, the high legitimacy messages seem to be the most effective of all the messages tested.
[…]
he coefficient plot in figure 4 shows the effect of sending any type of warning tweet on the ratio of tweets with hateful language over the tweets that a user tweets. The outcome variable is the ratio of hateful tweets over the total number of tweets that a user posted over the week and month following the treatment. The effects thus show the change in this ratio as a result of the treatment.
Figure 4 The effect of sending a warning tweet on reducing hateful language
Note: See table G1 in online appendix G for more details on sample size and control coefficients.
We find support for our first hypothesis: a tweet that warns a user of a potential suspension will lead that user to decrease their ratio of hateful tweets by 0.007 for a week after the treatment. Considering the fact that the average pre-treatment hateful tweet ratio is 0.07 in our sample, this means that a single warning tweet from a user with 100 followers reduced the use of hateful language by 10%.
[…]
The coefficient plot in figure 5 shows the effect of each treatment on the ratio of tweets with hateful language over the tweets that a user tweets. Although the differences across types are minor and thus caveats are warranted, the most effective treatment seems to be the high legitimacy tweet; the legitimacy category also has by far the largest difference between the high- and low-level versions of the three categories of treatment we assessed. Interestingly, the tweets emphasizing the cost of being suspended appear to be the least effective of the three categories; although the effects are in the correctly predicted direction, neither of the cost treatments alone are statistically distinguishable from null effects.
Figure 5 Reduction in hate speech by treatment type
Note: See table G2 in online appendix G for more details on sample size and control coefficients.
An alternative mechanism that could explain the similarity of effects across treatments—as well as the costliness channel apparently being the least effective—is that perhaps instead of deterring people, the warnings might have made them more reflective and attentive about their language use.
[…]
ur results show that only one warning tweet sent by an account with no more than 100 followers can decrease the ratio of tweets with hateful language by up to 10%, with some types of tweets (high legitimacy, emphasizing the legitimacy of the account sending the tweet) suggesting decreases of perhaps as high as 15%–20% in the week following treatment. Considering that we sent our tweets from accounts that have no more than 100 followers, the effects that we report here are conservative estimates, and could be more effective when sent from more popular accounts (Munger Reference Munger2017).
[…]
A recently burgeoning literature shows that online interventions can also decrease behaviors that could harm the other groups by tracking subjects’ behavior over social media. These works rely on online messages on Twitter that sanction the harmful behavior, and succeed in reducing hateful language (Munger Reference Munger2017; Siegel and Badaan Reference Siegel and Badaan2020), and mostly draw on identity politics when designing their sanctioning messages (Charnysh et al. Reference Charnysh, Lucas and Singh2015). We contribute to this recent line of research by showing that warning messages that are designed based on the literature of deterrence can lead to a meaningful decrease in the use of hateful language without leveraging identity dynamics.
[…]
Two options are worthy of discussion: relying on civil society or relying on Twitter. Our experiment was designed to mimic the former option, with our warnings mimicking non-Twitter employees acting on their own with the goal of reducing hate speech/protecting users from being suspended
[…]
hile it is certainly possible that an NGO or a similar entity could try to implement such a program, the more obvious solution would be to have Twitter itself implement the warnings.
[…]
the company reported “testing prompts in 2020 that encouraged people to pause and reconsider a potentially harmful or offensive reply—such as insults, strong language, or hateful remarks—before Tweeting it. Once prompted, people had an opportunity to take a moment and make edits, delete, or send the reply as is.”Footnote 15 This appears to result in 34% of those prompted electing either to review the Tweet before sending, or not to send the Tweet at all.
We note three differences from this endeavor. First, in our warnings, we try to reduce people’s hateful language after they employ hateful language, which is not the same thing as warning people before they employ hateful language. This is a noteworthy difference, which can be a topic for future research in terms of whether the dynamics of retrospective versus prospective warnings significantly differ from each other. Second, Twitter does not inform their users of the examples of suspensions that took place among the people that these users used to follow. Finally, we are making our data publicly available for re-analysis.
We stop short, however, of unambiguously recommending that Twitter simply implement the system we tested without further study because of two important caveats. First, one interesting feature of our findings is that across all of our tests (one week versus four weeks, different versions of the warning—figures 2 (in text) and A1(in the online appendix)) we never once get a positive effect for hate speech usage in the treatment group, let alone a statistically significant positive coefficient, which would have suggested a potential backlash effect whereby the warnings led people to become more hateful. We are reassured by this finding but do think it is an open question whether a warning from Twitter—a large powerful corporation and the owner of the platform—might provoke a different reaction. We obviously could not test for this possibility on our own, and thus we would urge Twitter to conduct its own testing to confirm that our finding about the lack of a backlash continues to hold when the message comes from the platform itself.Footnote 16
The second caveat concerns the possibility of Twitter making mistakes when implementing its suspension policies.
[…]
Despite these caveats, our findings suggest that hate-speech moderations can be effective without priming the salience of the target users’ identity. Explicitly testing the effectiveness of identity versus non-identity motivated interventions will be an important subject for future research.
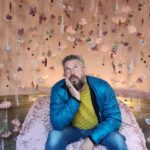
Robin Edgar
Organisational Structures | Technology and Science | Military, IT and Lifestyle consultancy | Social, Broadcast & Cross Media | Flying aircraft