Astrobiologists are mostly interested in rocky exoplanets that lie in the habitable zone around their parent stars, where liquid water may exist on its surface. NASA’s Kepler spacecraft has spotted a handful of these in the so-called Goldilocks Zone – where it’s not too cold or too hot for life.
As such, a second team from Google and NASA’s lab has built a machine-learning-based tool known as INARA that can identify the chemical compounds in a rocky exoplanet’s atmosphere by studying its high-resolution telescope images.
To develop this software, the brainiacs simulated more than three million planets’ spectral signatures – fingerprints of their atmospheres’ chemical makeups – and labelled them as such to train a convolutional neural network (CNN). The CNN can therefore be used to automatically estimate the chemical composition of a planet from images and light curves of its atmosphere taken from NASA’s Kepler spacecraft. Basically, a neural network was trained to link telescope images to chemical compositions, and thus, you should it a given set of images, and it will spit out the associated chemical components – which can be used to assess whether those would lead to life bursting on the scene.
INARA takes seconds to figure out the biological compounds potentially present in a world’s atmosphere. “Given the scale of the datasets produced by the Kepler telescopes, and the even greater volume of data that will return to Earth from the soon-to-be-launched Transiting Exoplanet Survey Satellite (TESS) satellite, minimizing analysis time per planet can accelerate this research and ensure we don’t miss any viable candidates,” Mascaro concluded. ®
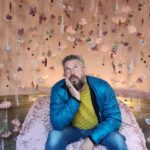
Robin Edgar
Organisational Structures | Technology and Science | Military, IT and Lifestyle consultancy | Social, Broadcast & Cross Media | Flying aircraft