Back in January, Google Health, the branch of Google focused on health-related research, clinical tools, and partnerships for health care services, released an AI model trained on over 90,000 mammogram X-rays that the company said achieved better results than human radiologists. Google claimed that the algorithm could recognize more false negatives — the kind of images that look normal but contain breast cancer — than previous work, but some clinicians, data scientists, and engineers take issue with that statement. In a rebuttal published today in the journal Nature, over 19 coauthors affiliated with McGill University, the City University of New York (CUNY), Harvard University, and Stanford University said that the lack of detailed methods and code in Google’s research “undermines its scientific value.”
Science in general has a reproducibility problem — a 2016 poll of 1,500 scientists reported that 70% of them had tried but failed to reproduce at least one other scientist’s experiment — but it’s particularly acute in the AI field. At ICML 2019, 30% of authors failed to submit their code with their papers by the start of the conference. Studies often provide benchmark results in lieu of source code, which becomes problematic when the thoroughness of the benchmarks comes into question. One recent report found that 60% to 70% of answers given by natural language processing models were embedded somewhere in the benchmark training sets, indicating that the models were often simply memorizing answers. Another study — a meta-analysis of over 3,000 AI papers — found that metrics used to benchmark AI and machine learning models tended to be inconsistent, irregularly tracked, and not particularly informative.
In their rebuttal, the coauthors of the Nature commentary point out that Google’s breast cancer model research lacks details, including a description of model development as well as the data processing and training pipelines used. Google omitted the definition of several hyperparameters for the model’s architecture (the variables used by the model to make diagnostic predictions), and it also didn’t disclose the variables used to augment the dataset on which the model was trained. This could “significantly” affect performance, the Nature coauthors claim; for instance, it’s possible that one of the data augmentations Google used resulted in multiple instances of the same patient, biasing the final results.
[…]
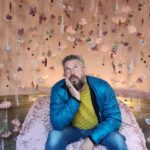
Robin Edgar
Organisational Structures | Technology and Science | Military, IT and Lifestyle consultancy | Social, Broadcast & Cross Media | Flying aircraft